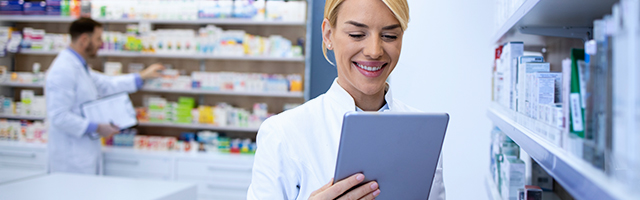
Scriptly Helps Pharmacies Identify Trends in Real Time with Reveal
Predictive analytics in healthcare refers to the analysis of current and historical healthcare data that allows healthcare professionals to find opportunities to make more effective and more efficient operational and clinical decisions, predict trends, and even manage the spread of diseases.
Healthcare, like any other industry today, is changing.
The abundance of data available at an organization’s fingertips transforms the entire industry. The way that diseases are discovered, how effectively patients are treated, and, even how hospitals utilize their resources so that care teams can coordinate and perform.
Real-time, accurate insights that can impact patients are extremely important.
And this is where healthcare analytics tools come to help.
Using predictive analytics in healthcare helps healthcare organizations, hospitals, and doctors to access, analyze, and process patient data to provide data-based quality care, accurate diagnosis, and personalized treatments.
Predictive analytics is a discipline in the data analytics world that relies heavily on modeling, data mining, AI, and machine learning techniques. It is used to evaluate historical and real-time data to make predictions about the future.
Predictive analytics in healthcare refers to the analysis of current and historical healthcare data that allows healthcare professionals to find opportunities to make more effective and more efficient operational and clinical decisions, predict trends, and even manage the spread of diseases.
Healthcare data is any data related to the health conditions of an individual or a group of people and is collected from administrative and medical records, health surveys, disease and patient registries, claims-based datasets, and EHRs. Healthcare analytics is a tool that anyone in the healthcare industry can use and benefit from to provide better-quality care – healthcare organizations, hospitals, doctors, physicians, psychologists, pharmacists, pharmaceutical companies, and even healthcare stakeholders.
The healthcare industry generates a tremendous amount of data but struggles to convert that data into useful insights to improve patient outcomes. Data analytics in healthcare is intended to be applied to every aspect of patient care and operations management. It is used to investigate methods of improving patient care, predicting disease outbreaks, reducing the cost of treatment, and so much more. At a business level, with the help of analytics, healthcare organizations can simplify internal operations, polish the utilization of their resources, and improve care teams’ coordination and efficiency.
The ability of data analytics to transform raw healthcare data into actionable insights has a significant impact in the following healthcare areas:
With technological advancement, analytics can tremendously impact the healthcare industry. AI and machine learning techniques can use data to diagnose diseases, determine the best treatment for each patient’s use case, and much more. Here are the most important ways in which healthcare organizations can benefit from predictive analytics:
The most important benefit that predictive analytics brings to the healthcare industry is the accessibility to all types of data – medical history, demographics, economics, and comorbidities. All this data provides doctors and healthcare professionals with valuable insights that guide their decisions. Better, smarter, and data-driven decisions lead to overall improved patient care.
For example, predictive analytics is used to improve patient outcomes. By looking at data and outcomes of old patients, machine learning algorithms can be programmed to provide insights into methods of treatment that will work best for each unique patient.
Traditionally, medicine has worked on a one-size-fits-all approach. Treatments and drugs have been prescribed based on limited information based on statistics of a broad population rather than specific patients. However, as medical professionals can more accurately diagnose patients, they can determine the most effective course of treatment tailored to the patient’s unique health situation.
Predictive analytics isn’t only applicable at the individual level. Healthcare organizations can use it also for population health management. When they have data about patients’ conditions, medications, and personal history, analytics can be used to find similar patients within a population cohort. It can also help identify cohorts exposed to a possible disease outbreak. In such a scenario, healthcare professionals can start looking at treatments immediately, which improves people’s chances of survival.
Predictive analytics in healthcare can predict which patients are at a higher risk and start early innervations so deeper problems can be avoided. For example, it can identify patients with cardiovascular disease with the highest probability of hospitalization based on age-coexisting chronic illnesses and medication adherence. Predictions on the likelihood of disease and chronic illness can help doctors and healthcare organizations proactively provide care rather than waiting for at-risk patients to come in for a regular checkup.
Besides the chronically ill patients, there are other at-risk groups, including elderly people and patients who have been recently discharged from the hospital after invasive manipulations.
Chronic diseases are the leading causes of death and disability in the US, as well as the main drivers of the country’s $3.5 trillion in annual health costs. Five chronic diseases account for 75% of healthcare spending: cancer, cardiovascular disease, diabetes, obesity, and kidney disease.
Chronic disease management depends on the ability of healthcare professionals to prevent the development of these diseases and to control them. Managing and preventing chronic conditions, however, is a challenging task. Predictive analytics can empower healthcare providers to make timely and fact-based informed decisions to provide more effective treatments while reducing the costs of this care to patients.
In other industries, such as manufacturing and telecommunication, predictive analytics has long been used to identify maintenance needs before they occur. The healthcare industry can benefit from the same kind of prognostics. Certain components of machines wear out or degrade. For example, by analyzing the data from sensors in an MRI machine, predictive analytics can predict failures and when a component will need to be replaced. By knowing that, hospitals can schedule maintenance at a time when the machine is not in use, minimizing workflow disruption that hinders both care teams and patients.
The digitalization of health services completely transforms the way that patients and health professionals interact with each other. Nowadays, we can attach devices to our bodies and track our health and body performance at any given time from our mobile phones. For example, diabetics can monitor the rise in blood sugar at any moment without the need for finger pricks.
The impact that human errors could have on healthcare could be fatal. Thankfully, by providing real-time, accurate insights to guide medical professionals’ actions, data can help flag potential errors and prevent fatal mistakes.
Unfortunately, fraud in healthcare is a common problem. Fraudulent healthcare schemes come in many forms: individuals obtaining subsidized or fully-covered prescription pills that are actually unneeded and then selling them on the black market for a profit; billing for a non-covered service as a covered service; modifying medical records; intentional incorrect reporting of diagnoses or procedures to maximize payment; prescribing additional or unnecessary treatment, etc.
Predictive analytics can identify certain abnormalities that flag these fraudulent actions, thus helping catch on to them early on.
Predictive analytics can also be used to reduce healthcare costs. It can be used to cut patient costs by reducing unnecessary care of hospitalization when it is not needed, controlling hospital costs of drugs and supplies, and predicting hospital staffing needs.
Predictive modeling (often called predictive analytics) is a mathematical process that uses statistical methods, data mining, and machine learning to identify patterns in data and recognize the chance of particular outcomes occurring. The goal of predictive modeling is to answer this question: ‘’What is most likely to happen in the future based on known past behavior?’’
The predictive modeling process involves running algorithms on data for prediction. Because the process is iterative, it trains the model that is most applicable for the goal or business fulfillment. The predictive modeling process goes through the following analytical modeling stages:
Predictive modeling in healthcare helps to improve patient care and ensure favorable outcomes. It can identify the highest-risk patients in poor health that will benefit most from intervention. Moreover, predictive analytics delves into insights from patterns in inpatient data in order to develop effective campaigns, predict product safety and optimize dosing, inform clinical trial designs, and much more.
In 2018, the average adult readmission rate reached 14%, with 20% of them referring to one of four conditions – diabetes, heart failure, COPD, and septicemia. With the use of socioeconomic data, EHRs, and predictive analytics, patients with a high risk of readmission can be discovered, warned, and provided additional medical care to reduce readmission rates.
Predictive analytics can be as effectively used in the research of new treatments too. The predictive algorithms can accurately predict the person’s response to a medication or treatment plan based on genetic information, clinical history, and other data. This can streamline the research process and cut the need inpatient for groups.
Another use of predictive analytics in healthcare is the ability to calculate the accurate cost of health insurance for each specific individual based on age, gender, medical history, insurance case history, heredity, etc. More so, predictive analytics can be used to prevent fraudulent insurance claims.
The National Healthcare Anti-Fraud Association estimates that the financial losses due to healthcare fraud amount from 3% up to 10% of funds spent on healthcare (this is equivalent to $300 billion). With the help of predictive analytics, insurance companies can develop and train ML algorithms to determine whether there is any malicious intent behind the case early on, thus reducing the losses and preventing future scamming attempts.
Reveal is embedded analytics software that helps unlock the power of data across health systems and datasets with a robust healthcare data analytics solution. Reveal’s advanced analytics provides healthcare organizations with a real-time, contextual view of their data, assisting healthcare professionals to deliver better care by empowering them to make smarter and data-driven decisions.
Integrated seamlessly within Reveal’s powerful suite of features encompassing a true SDK, white label capability, and interactive visualizations, predictive analytics stands as a cornerstone, leveraging machine learning models sourced from Azure ML and Google BigQuery. By harnessing this synergy, Reveal streamlines the data analysis process, empowering healthcare professionals with a comprehensive, real-time understanding of their patients’ data.
Reveal also comes built with many other robust analytics features, including intuitive drag-and-drop functionality for a simple dashboard creation experience, data blending, calculated fields, statistical functions, dashboard linking, drill-down, interactive chart filtering, and more. We also provide dashboard templates and the option to create your own custom data visualizations with just a few lines of code.
With the help of Reveal’s modern healthcare dashboard and healthcare analytics, you can track and monitor patients’ metrics, diagnostic trends, and more to gain deeper insights into people’s health and hospital performance.
If you’re interested in learning more about Reveal and how it can help you provide data-based quality care, accurate diagnosis, and personalized treatments, you can schedule a demo with one of our analytics experts or download our SDK to test the product yourself.
Back to Top